Usage of Artificial Intelligence in Stock Market Prediction
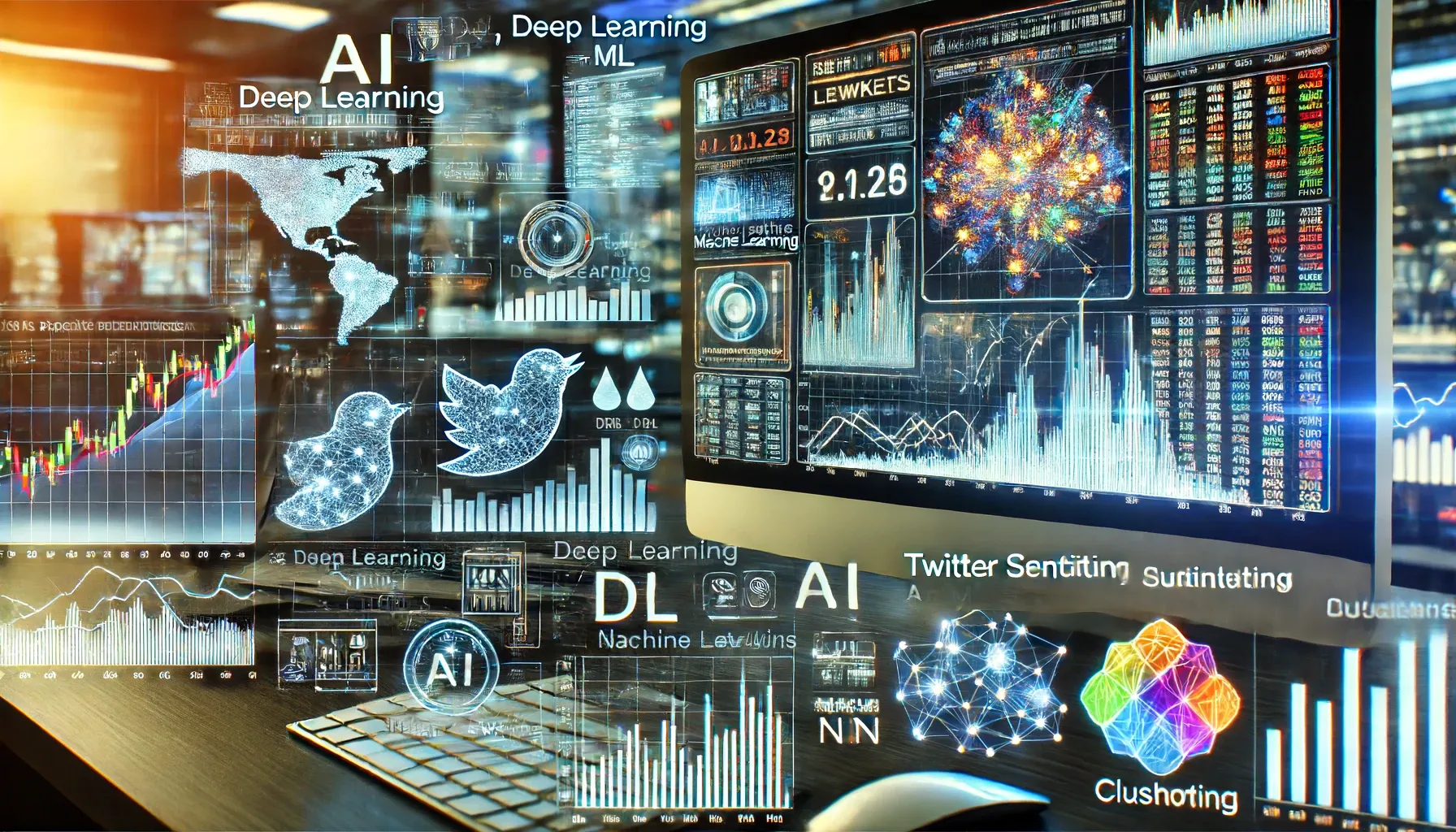
Context
The prediction of stock market is a challenging task. The price development of stocks is a complex system influenced by a lot of different forces like economic indicators, company financials or even global events. In the last years the world of technology is changing fast. The processing power of computers is improving exponentially, data is the new gold and is increasingly available online. While AI has long been a known term for people in the field of data science, the latest Large Language Models (LLM) such as OpenAI ChatGPT, Alphabet Gemini and Microsoft Copilot have made AI a household name for almost everyone. The development of Machine Learning (ML) and Deep Learning (DL) is creating new opportunities to use big amounts of data and computing power.
Objective
The objective of this thesis is about the use of these new technologies, such as certain ML or DL models, to test the extent to which predictions are possible in the stock market by using historical financial data. And use the possibility to access the big amount of data which is available on different social media platforms to see if the people in this world can influence or give information about the future price development of a company.
Method
The methodology involved different steps. First analyzing historical financial data of Nvidia. Developing and testing various classification models. Enhance these analyses by adding more predicting information, like using other stocks with similar price development as Nvidia and sentiment gained from social media platform regarding the Nvidia company.
Results
Historical financial data holds a lot of information about the price development of a stock. By analyzing the historical trends, different ratios, and the close values over time the neural network was the best performing model with a accuracy of 54%. Even though the accuracy is better than a random 50/50 prediction is is not really usable in a real world scenario, because there are a lot of false positive values, indicating a stock to go up when in reality it doesn't. This is the worst case scenario, when investing money based on a prediction.
By adding more information from sentiment analysis (SA) of social media posts from the platform "X", the models aimed to incorporate public opinion and social influence into the predictions. The SA was able to accurately categorize posts as positive or negative. However, the impact of these sentiments on stock predictions was minimal. While there is a potential for sentiment data to enhance prediction models, the results indicated that the current models require much more data to effectively learn and predict stock movements.
In a third approach more historical financial data of similar stocks was added as predictive features. With the clustering and correlation analysis it was possible to get companies with a similar price development. These information from the stock was not helping to achieve better results on the prediction of the Nvidia stock. But it could be used else, for example when creating a stock market portfolio to achieve a high diversity of the stocks and separate similar or opposite performing stock companies.